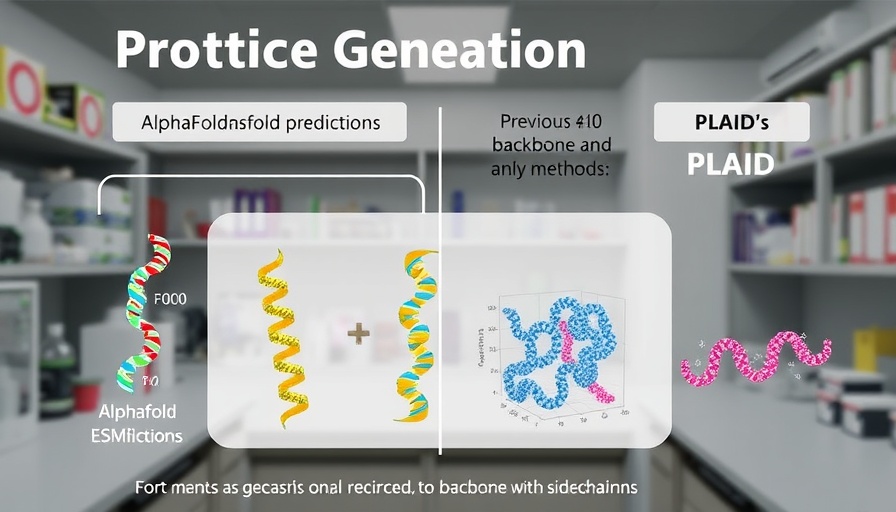
Understanding PLAID: The Future of Protein Generation
The recent awarding of the 2024 Nobel Prize to AlphaFold2 highlighted the transformative role of artificial intelligence in the realm of biological sciences. As we marvel at this achievement, an essential question looms: what's next for AI and protein folding? Enter PLAID, an innovative model that leverages latent diffusion to generate both the 1D amino acid sequence and the 3D structure of proteins.
Unpacking the Power of Latent Diffusion
PLAID stands out from traditional generative models by solving the multimodal co-generation problem. Unlike many predecessors that focus solely on backbone atoms, PLAID's ability to generate all-atom structures while simultaneously handling sequence data offers a significant advantage. This dual capability is crucial for tasks like drug design, where having complete and accurate protein structures can enhance efficacy.
Bridging the Gap Between Sequence and Structure
One of the challenges facing proteomics is the reliance on structural data, which are often scarce and expensive to obtain. PLAID circumvents this by utilizing vast sequence databases—2-4 orders of magnitude larger than their structural counterparts. By training only on sequences, PLAID builds a robust generative model that learns critical biological patterns without necessitating extensive structural data.
Humanizing Proteins for Enhanced Efficacy
The specificity of proteins intended for human therapeutic use must be addressed, as biologics can induce immune responses if not properly humanized. PLAID's architecture includes organism prompts to ensure that the generated proteins are suitable for human applications. This recognition of organism specificity marks a significant step from traditional models, broadening the utility of AI in biotech.
Controls and Constraints: The Path from Concept to Application
Generating proteins is only half the battle. The ability to specify complex constraints—such as solubility or structural requirements—is paramount for practical applications. PLAID takes inspiration from compositional prompts in image generation, creating an interactive interface where users can dictate parameters for protein functions. This evolution paves the way for more targeted drug discovery processes.
The Bigger Picture: AI’s Role Beyond Protein Folding
As CEOs and CMOs explore the potential of AI for transforming their organizations, understanding advancements like PLAID can inform strategic decisions in healthcare innovation and product development. The intersection of AI with biotechnology doesn't just hold promise for more effective drugs but also has implications for cost reduction and efficiency in research and development.
In conclusion, as AI continues to bridge previously insurmountable gaps in biology, tools like PLAID may well lead us to the next generation of therapeutics, fostering innovation across the biotech landscape. The ability to generate protein sequences that fulfill specific clinical roles can expedite drug discovery processes, making it an area worth exploring for organizational leaders in biopharma.
If you are leading an organization considering the integration of AI solutions in biotech, don't miss out on understanding how innovations like PLAID can drive efficiency and effectiveness in your workflows. Reach out to thought leaders and technology providers to better understand how to leverage these generative models for your unique challenges.
Write A Comment