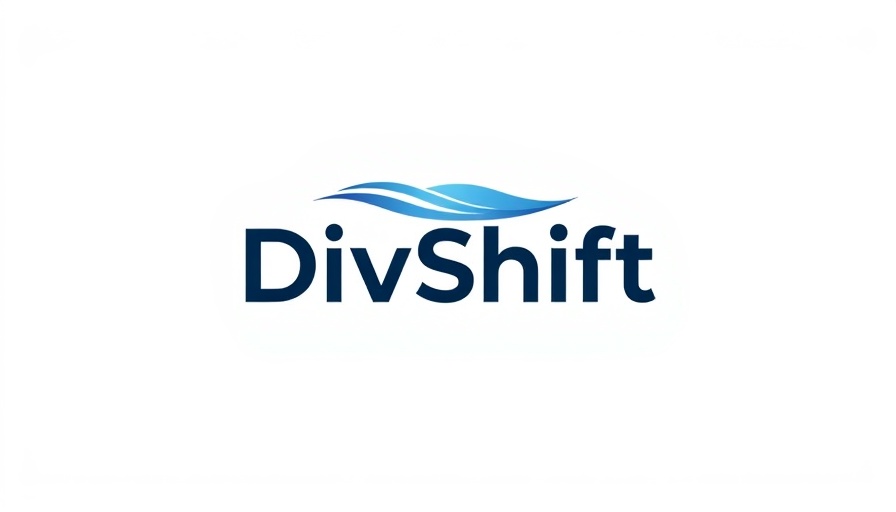
Understanding the Bias in Citizen Science Data
As AI technologies become increasingly integrated into biodiversity research, the need to address biases in data collected from citizen science platforms emerges as a critical issue. Platforms like iNaturalist surmount the traditional constraints of scientific research by allowing everyday citizens to contribute valuable data about biodiversity. However, this opportunistic data collection inherently introduces several biases.
Spatial biases, for instance, arise when certain geographic areas—often urban or popular nature spots—are over-represented. Temporal biases can also occur, with species observed during specific seasons getting disproportionately recorded. Further complicating this picture are taxonomic and observer behavior biases, all contributing to a skewed understanding of biodiversity. This reality must be acknowledged, as neglecting it can lead AI models to misrepresent ecological phenomena and misguide conservation efforts.
Introducing DivShift: A New Framework for Understanding Bias
The recently proposed DivShift framework tackles the challenge of quantifying these biases and their impacts on deep learning model performances used in biodiversity research. At its core, DivShift treats biases not as nuisances but as crucial “domain-specific distribution shifts.” This perspective allows for a systematic analysis of how these biases demonstrate varying impacts on model accuracy.
The framework leverages a novel dataset known as DivShift-NAWC, which comprises approximately 7.5 million plant images gathered from iNaturalist. By partitioning this extensive data along five documented axes of bias, DivShift enables researchers to compare model performances across previously underrepresented or misrepresented biodiversity segments, offering insights critical for training AI models appropriately.
The Implications for AI Model Training and Ecology
For leaders in technology and ecology, understanding how these biases impact AI model performance is invaluable. The DivShift framework not only quantifies the accuracy of models trained on biased data but also helps predict how these models may falter in less-sampled regions or for less-charismatic species. Accordingly, organizations focused on environmental sustainability and biodiversity conservation should prioritize frameworks like DivShift to ensure that their efforts are grounded in accurate and representative data.
In doing so, businesses can align AI solutions with ethical principles and conservation-focused goals, ultimately fostering a responsible organizational transformation through technology.
The Future of Biodiversity Data and AI
Looking ahead, we can anticipate that tools such as DivShift will play a pivotal role in shaping how biodiversity datasets are utilized in AI model training. As businesses and organizations in the biotechnology and sustainability sectors navigate the complexities of citizen science data, the need for robust methodologies that address underlying biases will become increasingly prominent.
Furthermore, the successful implementation of these methodologies can serve as a blueprint for more advanced AI applications, ensuring that they are built on sound ecological principles and adequately represent the realities of biodiversity. For CEOs, CMOs, and COOs, this is not merely an academic exercise; it is a calls to action to harness AI responsibly and effectively in their operations, paving the way for significant advancements in both ecological research and business integrity.
Write A Comment