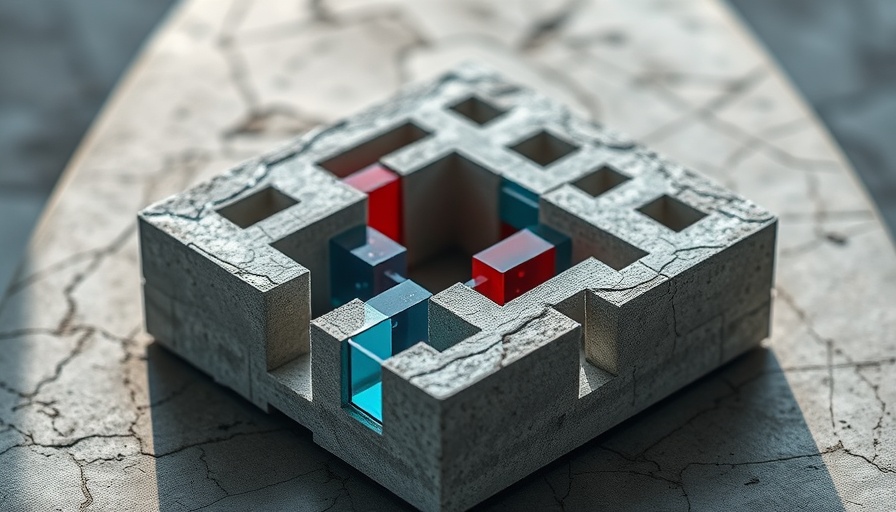
Navigating the Complexities of AI Agents: Autonomy and Safeguards
As AI becomes an integral part of business transformation strategies, understanding the intricacies of AI agents is crucial for executives and decision-makers. Notably, AI agents hold immense potential to enhance enterprise efficiency by iteratively refining tasks and fostering communication across departments. Yet, the promise of automation is accompanied by the imperative to establish robust safeguards that ensure operational safety and compliance.
Key Safeguards for Autonomous Operations
The allure of autonomous AI agents is significant, but autonomy without oversight can lead to significant operational risks. To mitigate potential errors or legal exposure, it's essential to implement various levels of safeguards tailored to each agent's role. For instance, integrating a set of predefined rules to trigger human intervention can prevent unchecked agent activities and increase confidence in their outputs. A purchasing agent, for example, should rely on human verification before proceeding with any transaction.
Embracing Uncertainty in AI Outputs
AI agents, particularly those relying on large language models (LLMs), may produce results with varying degrees of certainty. To address this, a method to gauge the uncertainty of outputs can be employed. By generating multiple outputs for ranking, agents can prioritize the most reliable results, albeit at a potential cost to speed and resources. Carefully considering this trade-off is vital for critical applications where reliability is paramount.
Future Trends in AI Agent Integration
Looking ahead, the integration of AI agents promises not only enhanced productivity but also new challenges and opportunities. Developing a robust agent network involves iterative refinement and adaptation, emphasizing dynamic systems capable of evolving with technological advancements. Adapting to these trends will allow enterprises to harness AI's full potential while being prepared for any emerging challenges.
Practical Tips for Implementation
For a seamless integration process, consider starting with less critical agents to establish a reliable operational foundation. This can be achieved by employing a report or work-order system that allows agents to suggest manual actions as initial steps. Such a strategy provides a controlled environment to debug and iterate, offering a strong baseline for scaling AI agent operations.
Write A Comment