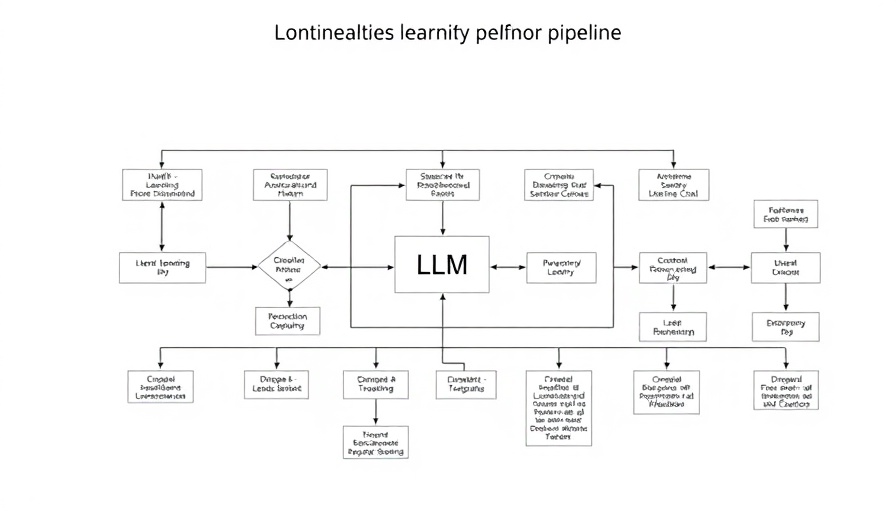
The Future of AI: Continuous Self-Instruct Fine-Tuning on AWS
In an era where decision-making at the executive level blends with cutting-edge technology, the application of continuous self-instruct fine-tuning for large language models (LLMs) is revolutionizing organizational transformation. Designed on the robust infrastructure of Amazon SageMaker, this innovative framework ensures that businesses remain agile in a constantly changing landscape by enabling their AI systems to adapt and improve continuously.
Fine-Tuning LLMs for Real-World Application
Continuous fine-tuning emerges as a critical approach that enhances LLM performance, enabling them to adapt to evolving datasets and maintain relevance. As highlighted in the AWS blog, this process effectively addresses concept drift—a phenomenon where the statistical properties of the target variable change over time. For leaders—especially CEOs, CMOs, and COOs—this approach can prove invaluable in ensuring that AI investment continues to yield tangible results.
Streamlining the Fine-Tuning Process
The self-instruct fine-tuning framework expedites the intricate workflow of AI model training, synthesizing human feedback along the way. By utilizing human-annotated datasets, businesses can leverage supervised fine-tuning (SFT) to more closely align their AI outputs with user preferences. This ongoing interaction promotes the model's learning trajectory, allowing it to deliver increasingly accurate and relevant responses in real time.
The Role of Compound AI Systems
A key component of this framework is the transition from traditional predictive models to compound AI systems. As noted in previous discussions within the industry, compound systems combine multiple interacting AI components instead of relying solely on monolithic models. This integration significantly enhances model performance and serves to cultivate innovation across various organizational sectors.
Benefits of Continuous Learning and Adaptation
The continuous self-instruct framework not only boosts model performance but also increases the versatility of AI applications. By employing tools like DSPy, businesses can automate the prompt tuning process, allowing for efficient iteration and optimization. By incorporating methods such as reinforcement learning from human feedback (RLHF) and preference alignment techniques, executives can harness the power of AI to drive forward-thinking strategies.
Case Studies and Real-World Applications
Take, for example, a large enterprise invested in conversational AI. By implementing a framework similar to the one described, they could improve customer interactions through enhanced question-answering capabilities. With the ability to generate synthetic datasets from existing knowledge bases, organizations can ensure their AI systems continuously learn and refine their responses based on client engagement, ultimately leading to improved customer satisfaction and loyalty.
Future Predictions and Insights
The trajectory of AI technology shows no signs of slowing, and as compound AI systems gain traction, leaders must proactively explore their potential benefits. As more executives recognize the value of AI-driven analytics in business strategy, those who adopt continuous self-instruct fine-tuning frameworks may find themselves ahead in operational efficiency and innovation.
In conclusion, leveraging a continuous self-instruct fine-tuning framework through Amazon SageMaker offers companies a crucial competitive advantage. As AI capabilities evolve, understanding and implementing these cutting-edge strategies will empower leaders across all industries to navigate complexities and respond effectively to changing market conditions.
Write A Comment