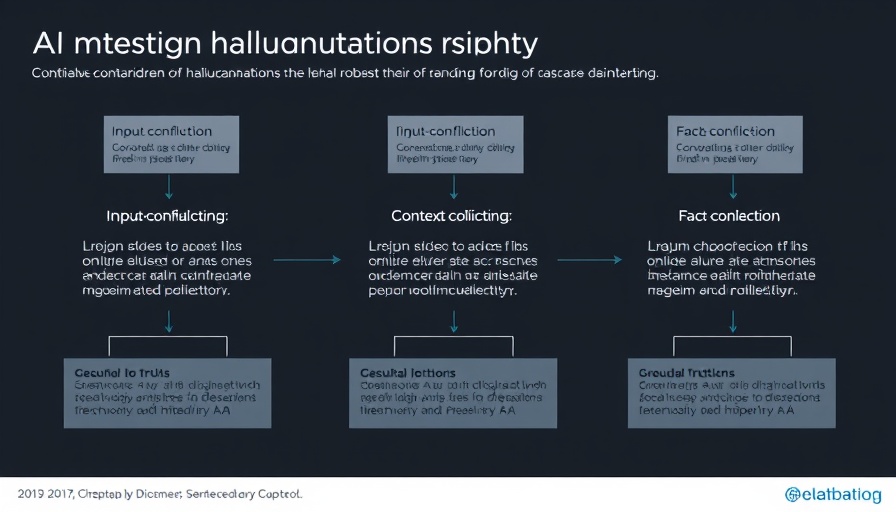
The Need for Accurate AI Systems in Business
In today’s rapidly evolving technological landscape, the integration of Artificial Intelligence (AI) into business operations is no longer a luxury but a necessity. As more organizations strive to leverage AI to enhance productivity and drive innovation, the accuracy and reliability of these AI systems become critical. One major challenge within AI, particularly in Natural Language Processing (NLP) applications, is the phenomenon known as 'hallucinations,' where AI generates information that is untrue or misleading. Understanding and managing these hallucinations is essential for businesses aiming to implement AI effectively.
Understanding AI Hallucinations: Why They Matter
Hallucinations in AI occur when models produce outputs that deviate from factual reliability, raising concerns about their application in critical business decision-making. These inaccuracies can erode trust among stakeholders and harm organizational reputation. As highlighted by AWS, identifying and mitigating hallucinations is particularly vital for Retrieval-Augmented Generation (RAG) systems that combine retrieval mechanisms with generative models for enhanced responses. For CEOs, CMOs, and COOs, comprehending the implications of such errors is fundamental to safeguarding their business interests.
Technology Innovations: The Role of RAG Systems
RAG systems are increasingly being adopted by organizations seeking to enhance their conversational AI capabilities. These systems utilize external data retrieval to inform their generative models, creating outputs that ideally reflect pertinent information. However, the risk of hallucinations persists. By recognizing triggers for hallucinations, such as ambiguous references or programmatic limitations, organizations can strategize around more accurate AI implementation, ensuring that their technology solutions remain credible.
Strategies to Mitigate Hallucinations in AI
To address the challenges posed by hallucinations, organizations can adopt several strategies:
- Robust Data Handling: Ensuring high-quality data is the foundation of reliable AI systems. Businesses should invest in data validation processes and maintain diverse data sources, reducing the likelihood of generating misleading outputs.
- Implementing Feedback Loops: Feedback mechanisms can be integrated into the AI’s training process, allowing machine learning models to learn from mistakes and improve over time.
- Regular Audits and Monitoring: Continuous evaluation of AI outputs will help identify patterns of hallucination, allowing for timely adjustments.
Future Predictions: Evolving Beyond Hallucinations
As AI technology continues to advance, the expectation is that systems will become increasingly capable of providing accurate results while minimizing hallucinations. Organizations should anticipate a shift towards more sophisticated algorithms and data structures designed to filter out potential inaccuracies. This evolution presents an opportunity for leaders to embrace a strategic role in AI governance and innovation, leading their businesses into a more data-driven future.
Conclusion: Embracing AI Responsibly
As AI permeates various aspects of organizational operations, understanding the nuances of hallucinations will empower leaders to harness these technologies more effectively. By adopting strategies that prioritize accuracy and trust, companies can confidently leverage AI while mitigating the risks associated with hallucinations. Embracing such knowledge not only brings improvements to operational efficiency but also redefines business ethics in the age of AI.
Write A Comment