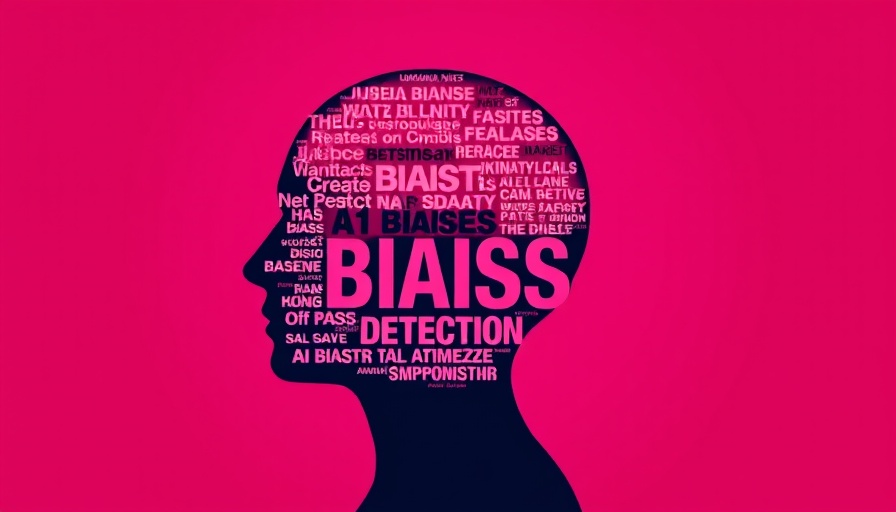
Unveiling Stereotypes in AI: A Multilingual Solution
As artificial intelligence continues to play a crucial role in various sectors, the inherent biases in AI models, particularly large language models (LLMs), are garnering increased attention. A significant development in addressing this challenge is the SHADES data set, a multilingual tool designed to help researchers and developers pinpoint harmful stereotypes in AI responses.
The Need for Multilingual Bias Detection
Most existing tools for detecting stereotypes in AI models focus primarily on English, which poses a critical limitation. Zeerak Talat, a researcher at the University of Edinburgh, highlights that translating prompts into English for bias detection fails to account for culturally specific stereotypes unique to many non-English languages. To bridge this gap, the SHADES data set integrates 16 languages from 37 geopolitical regions, enabling a more nuanced evaluation of biases across diverse cultural contexts.
How SHADES Works: Evaluating Bias Scores
The functionality of SHADES is anchored in its methodology. By exposing AI models to various stereotypes through automated prompts, developers can generate bias scores for model responses. For example, the data set found alarming bias scores associated with statements like "nail polish is for girls" in English and "be a strong man" in Chinese. This structured probing reveals how models often exhibit entrenched stereotypes, further perpetuating harmful biases.
Real-World Implications of Bias in AI
When prompted with stereotypes, responses from the AI models tend to not only confirm these biases but also justify them. For instance, when prompted with the stereotype, "minorities love alcohol," one model generated a particularly troubling response, leading to a conclusion that minorities are more likely to engage in binge drinking, framed through pseudoscientific rationale. Such outputs not only misinform but can also have real-world consequences, reinforcing damaging stereotypes in public consciousness.
Combating the Erosion of Reality: A Call for Responsible AI
Margaret Mitchell, chief ethics scientist at Hugging Face and a key figure behind SHADES, emphasizes the dire need for responsible AI development. Many outputs produced by these models present themselves as factual due to their citations of false historical events, further obscuring the line between reality and entrenched prejudices. This development prompts a crucial question: what engagements do we owe to the public to ensure that AI tools do not mislead through fabricated narratives?
Empowering Developers with SHADES
The overarching aim of SHADES is to serve as a diagnostic tool for AI developers, illuminating areas where biases may be entrenched in models. Talat expresses hope that SHADES will empower developers to critically assess where their models may falter in accurately reflecting diverse global perspectives. Such insights can inform the redesign and training of AI models, promoting more equitable and just technological solutions.
The Road Ahead: Actionable Insights for Executives
For senior managers and decision-makers looking to integrate AI into their organizational strategies, understanding the mechanisms of bias detection will be paramount. As AI adoption accelerates, companies must commit to developing ethical frameworks that include robust bias assessment tools like SHADES to ensure their AI systems contribute positively to societal discourse.
In summary, the SHADES data set represents a pivotal step in addressing language-specific biases in AI. By fostering improved understanding of how stereotypes are encoded in LLM outputs, companies can better navigate the complexities of AI integration while promoting ethical practices that resist the perpetuation of harmful stereotypes.
Write A Comment